Home Publications edited volumes Awards Research Teaching Miscellaneous Full CV [pdf] BLOG bio
Events
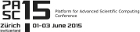
Past Events
|
Publications of Torsten Hoefler
Peter Grönquist, Tal Ben-Nun, Nikoli Dryden, Peter Dueben, Luca Lavarini, Shigang Li, Torsten Hoefler:
| | Predicting Weather Uncertainty with Deep Convnets
(In Machine Learning and the Physical Sciences Workshop at the 33rd Conference on Neural Information Processing Systems (NeurIPS), presented in Vancouver, BC, Canada, Dec. 2019)
AbstractModern weather forecast models perform uncertainty quantification using ensemble prediction systems, which collect nonparametric statistics based on multiple perturbed simulations. To provide accurate estimation, dozens of such computationally intensive simulations must be run. We show that deep neural networks can be used on a small set of numerical weather simulations to estimate the spread of a weather forecast, significantly reducing computational cost. To train the system, we both modify the 3D U-Net architecture and explore models that incorporate temporal data. Our models serve as a starting point to improve uncertainty quantification in current real-time weather forecasting systems, which is vital for predicting extreme events.
Documents 
| | BibTeX | @article{, author={Peter Grönquist and Tal Ben-Nun and Nikoli Dryden and Peter Dueben and Luca Lavarini and Shigang Li and Torsten Hoefler}, title={{Predicting Weather Uncertainty with Deep Convnets}}, year={2019}, month={Dec.}, booktitle={Machine Learning and the Physical Sciences Workshop at the 33rd Conference on Neural Information Processing Systems (NeurIPS)}, location={Vancouver, BC, Canada}, source={http://www.unixer.de/~htor/publications/}, } |
|
|